Unlocking the Power of Image Annotation for Machine Learning
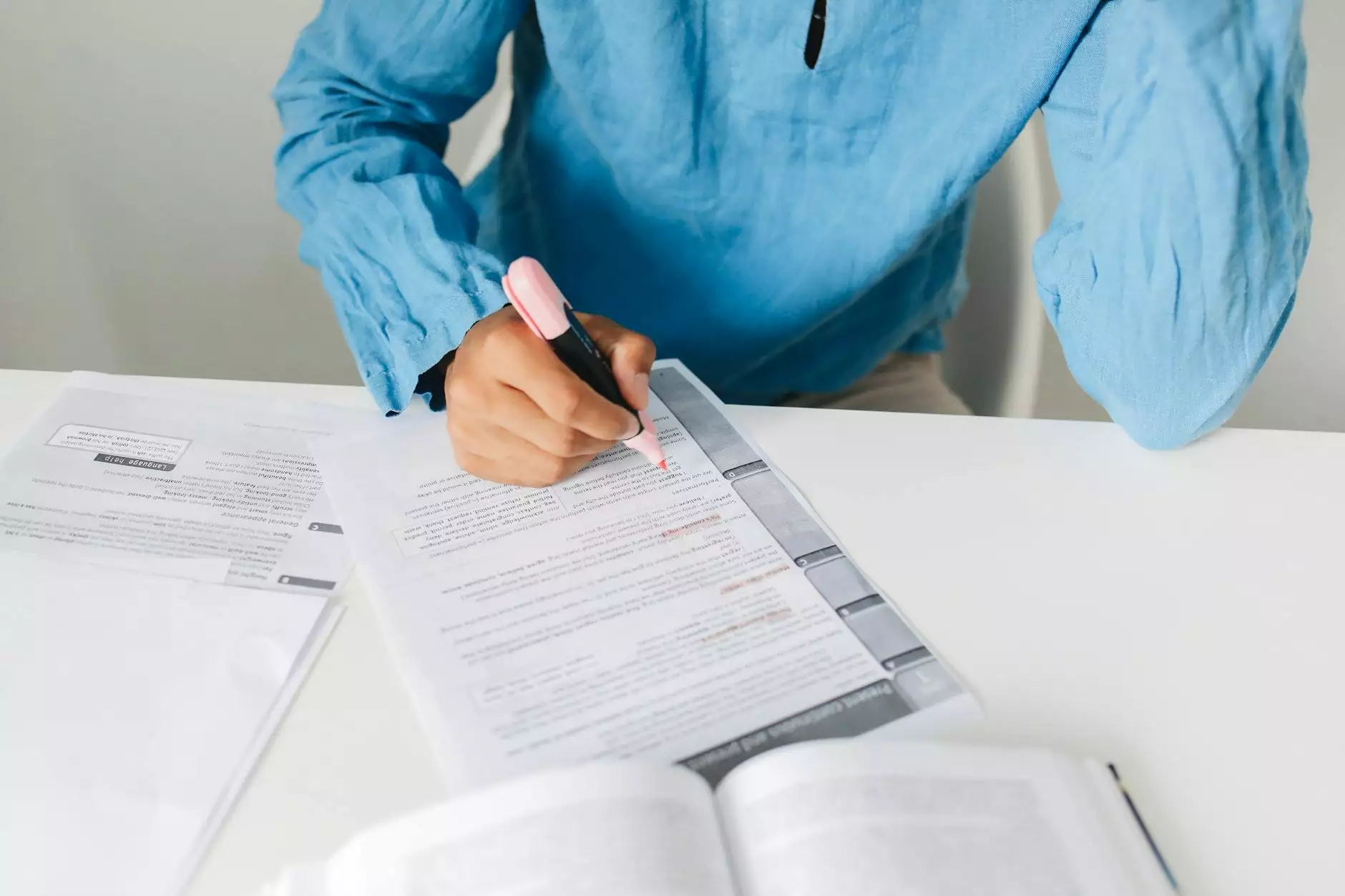
In today's rapidly evolving technological landscape, the need for high-quality data has become paramount, especially in the realm of machine learning. One critical component in this area is image annotation for machine learning. This article delves into the importance, methodologies, and tools associated with image annotation in enhancing machine learning algorithms.
The Importance of Image Annotation in Machine Learning
Machine learning models thrive on data. The more diverse and accurate the data, the better the model can learn and make predictions. Image annotation refers to the process of labeling images with metadata, allowing machines to interpret the visual content. This is particularly crucial when developing applications that rely on image recognition, such as autonomous vehicles, facial recognition systems, and Object Detection.
How Image Annotation Works
The image annotation process involves several steps, tailored to the specific needs of a project. These steps include:
- Data Collection: Gathering a diverse set of images that will be used for training the model.
- Labeling: Annotators label the images by drawing bounding boxes, polygons, or applying masks to define the objects within the images.
- Quality Assurance: Ensuring that annotations are accurate and consistent across the dataset, which is vital for training robust models.
- Integration: Feeding the annotated data into machine learning models to train them effectively.
Each of these steps contributes to the overall success of machine learning applications, making effective data annotation tools indispensable.
Diverse Applications of Image Annotation
Image annotation plays a critical role across various industries, each benefiting uniquely from this technology. Here are some prominent applications:
Autonomous Vehicles
Self-driving cars rely heavily on accurate image annotation to identify road signs, pedestrians, and other vehicles. This information is processed by neural networks that learn how to navigate safely in real-time environments.
Healthcare
In the medical field, image annotation is used to analyze medical images such as X-rays and MRIs. Annotated images help radiologists diagnose conditions more accurately and develop predictive models for patient outcomes.
Facial Recognition
Facial recognition technology utilizes image annotation to detect and identify human faces within images. This has applications in security systems, social media, and personal devices, enhancing user experience and safety.
Retail and E-commerce
In retail, image annotation is used to categorize products and help in search functionality. Annotated images improve user experience by providing more relevant search results to consumers.
Key Features of an Effective Data Annotation Tool
When selecting a data annotation tool for machine learning projects, it’s essential to consider several key features:
- User-Friendly Interface: A simple interface allows annotators to work efficiently without extensive training.
- Collaboration Features: Support for multiple users collaborating in real-time can significantly enhance productivity.
- Integration with Machine Learning Pipelines: The ability to seamlessly integrate with existing workflows is vital for maintaining efficiency.
- Support for Various Annotation Formats: Flexibility in choosing annotation types—bounding boxes, polygons, and segmentation masks—accommodates different project needs.
- Quality Control Mechanisms: Built-in quality assurance processes help ensure annotations meet accuracy standards.
Choosing the Right Data Annotation Platform
With numerous options available, selecting the right data annotation platform can be challenging. Here are some pointers to guide your decision:
Evaluate Your Project Requirements
Before choosing a platform, assess the specific needs of your project. Consider the types of images you will be working with, the volume of data, and the complexity of annotations required.
Assess Scalability
Choose a platform that can grow with your project. As your dataset expands, you will need a solution that can accommodate increased workloads without compromising quality.
Examine Support and Documentation
Look for platforms that offer robust customer support and extensive documentation. This will help you navigate any challenges that arise during the annotation process.
How Keylabs.ai Stands Out in Image Annotation
At keylabs.ai, we specialize in providing top-tier tools for image annotation for machine learning. Our platform is designed with both ease of use and advanced functionality in mind, ensuring that users can efficiently annotate images while maintaining high standards of quality.
Advanced Annotation Features
Keylabs.ai offers a variety of annotation options, including:
- Bounding Box Annotation
- Polygon Annotation
- Semantic Segmentation
- 3D Point Cloud Annotation
These features enable users to cater to various machine learning applications, ensuring that all data requirements are met.
Quality Assurance Protocols
Our platform incorporates stringent quality assurance protocols, which include:
- Automated Quality Checks
- Expert Review of Annotations
- Customizable Quality Metrics
These practices help maintain the integrity of the data, allowing machine learning models to be trained on the most accurate and reliable data possible.
Seamless Integration with ML Workflows
Keylabs.ai is specifically designed to integrate smoothly with your existing machine learning workflows. Whether you are using TensorFlow, PyTorch, or any other framework, our platform ensures a hassle-free transition from data annotation to model training.
The Future of Image Annotation in Machine Learning
As machine learning continues to grow, the importance of image annotation will become even more pronounced. Advances in artificial intelligence are leading to developments in automated annotation processes, where machine learning algorithms can begin to label images with minimal human intervention.
However, human oversight will remain crucial, particularly for complex or nuanced tasks where understanding context is essential. The balance between automation and human input will define the future of image annotation.
Preparing for Future Developments
To stay ahead in the rapidly changing landscape of machine learning, organizations must prioritize investment in advanced image annotation tools and processes. Collaborating with leading annotation platforms like keylabs.ai will equip you with the resources needed to excel.
Conclusion
In conclusion, image annotation for machine learning is a foundational element of successful machine learning projects. The accuracy and quality of annotated data directly impact the effectiveness of machine learning models. By leveraging advanced data annotation tools and adhering to best practices, organizations can unlock the full potential of their machine learning initiatives.
For those looking to elevate their annotation processes, Keylabs.ai stands out as a premier choice, combining user-friendly features with robust functionality. By investing in high-quality annotation solutions, you're not just improving your models; you're paving the way for innovative applications that can transform industries.